Happy to get feedback via @abhi_tweeter or just drop a comment!
One of the previous blogs was about building a stateless stream processing application using the Kafka Streams library and deploying it to Kubernetes as in the form of a Deployment
object.
In this part, we will continue exploring the powerful combination of Kafka Streams and Kubernetes. But this one is all about stateful applications and how to leverage specific Kubernetes primitives using a Kubernetes cluster on Azure (AKS) to run it.
I will admit right away that this is a slightly lengthy blog, but there are a lot of things to cover and learn!
As you go through this, you’ll learn about the following:
- Kafka Streams
- What is Kafka Streams?
- Concepts of stateful Kafka Streams applications
- Behind-the-scenes
- What’s going on in the Java code for stream processing logic using Kafka Streams
- Kubernetes components for running Stateful Kafka Streams apps such
StatefulSet
, Volume Claim templates and other configuration parameters like Pod
anti-affinity
- How is this all setup using Azure Kubernetes Service for container orchestration and Azure Disk for persistence storage
- How to set up and configure a Docker container registry and Azure Kubernetes cluster
- How to build & deploy our app to Kubernetes and finally test it out using the Kafka CLI
The source code is on GitHub
Let’s get started!
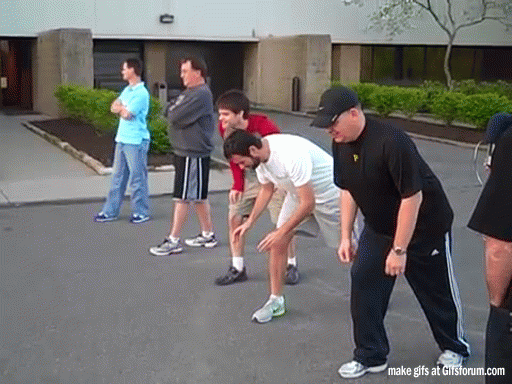
Pre-requisites:
If you don’t have it already, please install the Azure CLI and kubectl. The stream processing app is written in Java and uses Maven. You will also need Docker to build the app container image.
This tutorial assumes you have a Kafka cluster which is reachable from your Kubernetes cluster on Azure
Kafka Streams
This section will provide a quick overview of Kafka Streams and what “state” means in the context of Kafka Streams based applications.
Overview of Kafka Streams
It is a simple and lightweight client library, which can be easily embedded in any Java app or microservice, where the input and output data are stored in Kafka clusters. It has no external dependencies on systems other than Kafka itself and it’s partitioning model to horizontally scale processing while maintaining strong ordering guarantees. It has support for fault-tolerant local state, employs one-record-at-a-time processing to achieve millisecond processing latency and offers necessary stream processing primitives, along with a high-level Streams DSL and a low-level Processor API. The combination of “state stores” and Interactive queries allow you to leverage the state of your application from outside your application.
Stateful Kafka Streams app
Most stream processing apps need contextual data i.e. state in order e.g. to maintain a running count of items in an inventory, you’re going to need the last “count” in order to calculate the “current” count.
You can deploy multiple Kafka Streams app instances to scale your processing. Since each instance churns data from one or more partitions (of a Kafka topic), the state associated with each instance is stored locally (unless you’re the GlobalKTable
API – deserves a dedicated blog post!). Kafka Streams supports “stateful” processing with the help of state stores. Typically, it is file-system based (Kafka Streams uses an embedded RocksDB database internally) but you also have the option of using an in-memory hash-map, or use the pluggable nature of the Kafka Streams Processor API to build a custom implementation a state store.
In addition to storing the state, Kafka Streams has built-in mechanisms for fault-tolerance of these state stores. The contents of each state store are backed-up to a replicated, log-compacted Kafka topic. If any of your Kafka Streams app instance fails, another one can come up, restore the current state from Kafka and continue processing. In addition to storing state, you can also “query” these state stores. That’s a topic for another blog post altogether – stay tuned!
Please note that it is possible to tune the “fault tolerance” behavior i.e. you can choose not to back-up your local state store to Kafka
Before you dive in, here is a high level overview of the solution
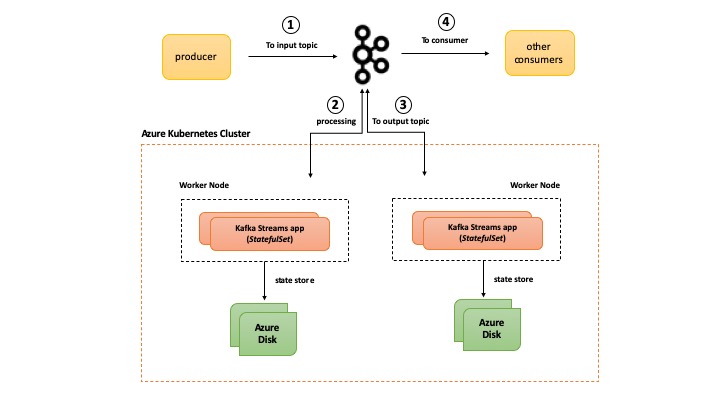
Behind the scenes
Let’s look at what the stream processing code is upto and then dive into some of the nitty-gritty of the Kubernetes primitives and what value they offer when running “stateful” Kafka Streams apps.
Stream processing code
The processing pipeline executes something similar to the canonical “word count”. It makes use of the high-level Streams DSL API:
- receives a stream of key-value pairs from an input/source Kafka topic e.g.
foo:bar
, john:doe
, foo:bazz
etc.
- keeps and stores a count of the keys (ignores the values) e.g.
foo=2
, john=1
etc.
- forwards the count to an output Kafka topic (sink)
Please note that the latest Kafka Streams library version at the time of writing was 2.3.0
and that’s what the app uses
org.apache.kafka
kafka-streams
2.3.0
We start off with an instance of StreamsBuilder
and invoke it’s stream
method to hook on to the source topic. What we get is a KStream
object which is a representation of the continuous stream of records sent to the topic.
StreamsBuilder builder = new StreamsBuilder();
KStream inputStream = builder.stream(INPUT_TOPIC);
We use groupByKey
on the input KStream
to group the records by their current key into a KGroupedStream
. In order to keep a count of the keys, we use the count
method (not a surprise!). We also ensure that the word count i.e. foo=5
, bar=3
etc. is persisted to a state store – Materialized
is used to describe how that state store should be persisted. In this case, a specific name is chosen and the exact location on disk is mentioned in the KafkaStreams configuration as such: configurations.put(StreamsConfig.STATE_DIR_CONFIG, "/data/count-store");
the default behavior is to store the state on disk using RocksDB unless configured differently
inputStream.groupByKey()
.count(Materialized.<String, Long, KeyValueStore>as("count-store")
.withKeySerde(Serdes.String())
.withValueSerde(Serdes.Long()))
Finally, for ease of demonstration, we convert the KTable
(created by count
) back to a KStream
using toStream
, convert the java.lang.Long
(that’s the count
data type) into a String
using mapValues
and pass on the result to an output topic. This just for easy consumption in the Kafka CLI so that you’re able to actually see the final count of each of the words.
.toStream()
.mapValues(new ValueMapper() {
@Override
public String apply(Long v) {
return String.valueOf(v);
}
})
.to(OUTPUT_TOPIC);
That’s all in terms of setting up the stream and defining the logic. We create a Topology
object using the build
method in StreamsBuilder
and use this object to create a KafkaStreams
instance which is a representation of our application itself. We start the stream processing using the start
method
Topology topology = builder.build();
KafkaStreams streamsApp = new KafkaStreams(topology, getKafkaStreamsConfig());
streamsApp.start();
The getKafkaStreamsConfig()
is just a helper method which creates a Properties
object which contains Kafka Streams specific configuration, including Kafka broker endpoint etc.
static Properties getKafkaStreamsConfig() {
String kafkaBroker = System.getenv().get(KAFKA_BROKER_ENV_VAR);
Properties configurations = new Properties();
configurations.put(StreamsConfig.BOOTSTRAP_SERVERS_CONFIG, kafkaBroker + ":9092");
configurations.put(StreamsConfig.APPLICATION_ID_CONFIG, APP_ID);
configurations.put(StreamsConfig.DEFAULT_KEY_SERDE_CLASS_CONFIG, Serdes.String().getClass().getName());
configurations.put(StreamsConfig.DEFAULT_VALUE_SERDE_CLASS_CONFIG, Serdes.String().getClass().getName());
configurations.put(StreamsConfig.REQUEST_TIMEOUT_MS_CONFIG, "20000");
configurations.put(StreamsConfig.RETRY_BACKOFF_MS_CONFIG, "500");
configurations.put(StreamsConfig.STATE_DIR_CONFIG, STATE_STORE_DIR);
return configurations;
}
Kubernetes primitives
So far so good! We have the Kafka Streams app churning out word counts and storing them. We can simply run this as a Kubernetes Deployment
(as demonstrated in the previous blog), but there are some benefits to be gained by using something called a StatefulSet
.
StatefulSet
is a topic that deserves a blog (or more!) by itself. The goal is not to teach you everything about Kubernetes StatefulSet
s in this blog, but provide enough background and demonstrate how its features can be leveraged for stateful Kafka Streams apps.
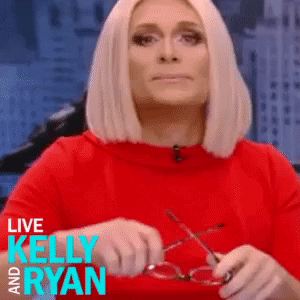
StatefulSet: the What
Here is a gist what StatefulSet
s offer for running stateful workloads in Kubernetes
Pod
uniqueness – Each Pod
in a StatefulSet
is unique and this maintained across restarts, re-scheduling etc. This also applies to networking and communication (inter-Pod or external)
- Persistent storage – using a Volume Claim template, you can request for storage allocation for each
Pod
in a StatefulSet
such that there is one to one mapping b/w the Pod
and the storage medium
- Managed lifecycle – You can be explicit about how to manage the lifecycle of the Pods across various stages including starts, updates, deletion.
StatefulSet
Pods can be configured to handle this is an ordered manner.
All these are in stark contrast to general Deployment
s which handle Pods as disposable entities with no identity, the concept of “stable” attached storage or ordered lifecycle management.
StatefulSet: the Why
Let’s explore the motivation behind why we want to use StatefulSet
s for this specific scenario i.e. a stateful Kafka Streams app.
As previously mentioned, you can run multiple Kafka Streams app instances. Each instance processes data from one or more partitions (of a topic) and stores the associated state locally. Fault tolerance and resiliency is also built into Kafka Streams app because the contents of each state store is backed-up to a replicated, log-compacted Kafka topic. If any of your Kafka Streams app instance fails, another one can come up, restore the current state from Kafka and continue processing.
Now here is the catch. Any serious application with a reasonably complex topology and processing pipeline will generate a lot of “state”. In such as case, regular app operations like scale-out or anomalies such as crashes etc. will trigger the process of restore/refresh state from the Kafka back-up topic. This can be costly in terms of time, network bandwidth etc. Using StatefulSet
, we can ensure that each Pod
will always have a stable storage medium attached to it and this will be stable (not change) over the lifetime of the StatefulSet
. This means that after restarts, upgrades etc. (most of) the state is already present locally on the disk and the app only needs to fetch the “delta” state from the Kafka topics (if needed). This implies that state recovery time will be much smaller or may not even be required in few cases.
In this example, we will be making use of the first two features of StatefulSet
i.e. Pod uniqueness and stable Persistent Storage.
StatefulSet: the How
It’s time to see how it’s done. Let’s start by exploring the Kubernetes YAML manifest (in small chunks) for our application – we will later use this to deploy the app to AKS
We define the name of our StatefulSet
(kstreams-count
) and refer to a Headless
Service(kstreams-count-service
) which is responsible for the unique network identity – it is bundled along with StatefulSet
itself.
apiVersion: apps/v1
kind: StatefulSet
metadata:
name: kstreams-count
spec:
serviceName: "kstreams-count-service"
The Headless
Service should be created before the StatefulSet
apiVersion: v1
kind: Service
metadata:
name: kstreams-count-service
labels:
app: kstreams-count
spec:
clusterIP: None
selector:
app: kstreams-count
The Pod
specification (spec.containers
) points to the Docker image and defines the environment variable KAFKA_BROKER
which which will be injected within our app at runtime.
spec:
containers:
- name: kstreams-count
image: .azurecr.io/kstreams-count:latest
env:
- name: KAFKA_BROKER
value: [to be filled]
In addition to the above, the container spec also defines the persistent storage. In this case, it means that the container will use a stable storage to store the contents in the specified path which in this case is /data/count-store
(recall that this is the local state directory as configured in our Kafka Streams app)
volumeMounts:
- name: count-store
mountPath: /data/count-store
How is this persistent storage going to come to life and made available to the Pod
? The answer lies in a Volume Claim Template specified as a part of the StatefulSet
spec. One PersistentVolumeClaim
and PersistentVolume
will be created for each Volume Claim Template.
volumeClaimTemplates:
- metadata:
name: count-store
spec:
accessModes: [ "ReadWriteOnce" ]
resources:
requests:
storage: 1Gi
So how does the storage medium get created?
This is powered by Dynamic Provisioning which enables storage volumes to be created on-demand. Otherwise, cluster administrators have to manually provision cloud based storage and then create equivalent PersistentVolume
objects in Kubernetes. Dynamic provisioning eliminates this by automatically provisioning storage when it is requested by users.
Dynamic provisioning itself uses a StorageClass
which provides a way to describe the type of storage using a set of parameters along with a volume plugin which actually takes care of the storage medium provisioning. Azure Kubernetes Service makes dynamic provisioning easy by including two pre-seeded storage classes:
You can check the same by running kubectl get storageclass
command
NAME PROVISIONER AGE
default (default) kubernetes.io/azure-disk 6d10h
managed-premium kubernetes.io/azure-disk 6d10h
Note that kubernetes.io/azure-disk
is the volume plugin (provisioner implementation)
Since, we don’t have an explicit StorageClass
defined in the volume claim template, so the default StorageClass
will be used. For each instance of your Kafka Streams app, an Azure Disk instance will be created and mounted into the Pod
representing the app.
Finally, we use Pod anti-affinity (nothing to do with StatefulSet
) – this is to ensure that no two instances of our app are located on the same node.
affinity:
podAntiAffinity:
requiredDuringSchedulingIgnoredDuringExecution:
- labelSelector:
matchExpressions:
- key: app
operator: In
values:
- kstreams-count
topologyKey: "kubernetes.io/hostname"
Let’s move on to the infrastructure setup.
AKS cluster setup
You need a single command to stand up a Kubernetes cluster on Azure. But, before that, we’ll have to create a resource group
export AZURE_SUBSCRIPTION_ID=[to be filled]
export AZURE_RESOURCE_GROUP=[to be filled]
export AZURE_REGION=[to be filled] (e.g. southeastasia)
Switch to your subscription and invoke az group create
az account set -s $AZURE_SUBSCRIPTION_ID
az group create -l $AZURE_REGION -n $AZURE_RESOURCE_GROUP
You can now invoke az aks create
to create the new cluster
To keep things simple, the below command creates a two node cluster. Feel free to change the specification as per your requirements
export AKS_CLUSTER_NAME=[to be filled]
az aks create --resource-group $AZURE_RESOURCE_GROUP --name $AKS_CLUSTER_NAME --node-count 2 --node-vm-size Standard_B2s --node-osdisk-size 30 --generate-ssh-keys
Get the AKS cluster credentials using az aks get-credentials
– as a result, kubectl
will now point to your new cluster. You can confirm the same
az aks get-credentials --resource-group $AZURE_RESOURCE_GROUP --name $AKS_CLUSTER_NAME
kubectl get nodes
If you are interested in learning Kubernetes and Containers using Azure, simply create a free account and get going! A good starting point is to use the quickstarts, tutorials and code samples in the documentation to familiarize yourself with the service. I also highly recommend checking out the 50 days Kubernetes Learning Path. Advanced users might want to refer to Kubernetes best practices or the watch some of the videos for demos, top features, and technical sessions.
Setup Azure Container Registry
Simply put, Azure Container Registry (ACR
in short) is a managed private Docker registry in the cloud which allows you to build, store, and manage images for all types of container deployments.
Start by creating an ACR instance
export ACR_NAME=[to be filled]
az acr create --resource-group $AZURE_RESOURCE_GROUP --name $ACR_NAME --sku Basic
valid SKU values – Basic
, Classic
, Premium
, Standard
. See command documentation
Configure ACR to work with AKS
To access images stored in ACR, you must grant the AKS service principal the correct rights to pull images from ACR.
Get the appId
of the service principal which is associated with your AKS cluster
AKS_SERVICE_PRINCIPAL_APPID=$(az aks show --name $AKS_CLUSTER_NAME --resource-group $AZURE_RESOURCE_GROUP --query servicePrincipalProfile.clientId -o tsv)
Find the ACR resource ID
ACR_RESOURCE_ID=$(az acr show --resource-group $AZURE_RESOURCE_GROUP --name $ACR_NAME --query "id" --output tsv)
Grant acrpull
permissions to AKS service principal
az role assignment create --assignee $AKS_SERVICE_PRINCIPAL_APPID --scope $ACR_RESOURCE_ID --role acrpull
For some more details on this topic, check out one of my previous blog
How to get your Kubernetes cluster service principal and use it to access other Azure services?
Alright, our AKS cluster along with ACR is ready to use!
From your laptop to a Docker Registry in the cloud
Clone the GitHub repo, change to the correct directory and build the application JAR
git clone https://github.com/abhirockzz/kafka-streams-stateful-kubernetes
cd kafka-streams-stateful-kubernetes
mvn clean install
You should see kstreams-count-statefulset-1.0.jar
in the target
directory
Here is the Dockerfile
for our stream processing app
FROM openjdk:8-jre
WORKDIR /
COPY target/kstreams-count-statefulset-1.0.jar /
CMD ["java", "-jar","kstreams-count-statefulset-1.0.jar"]
We will now build a Docker image …
export DOCKER_IMAGE=kstreams-count
export ACR_SERVER=$ACR_NAME.azurecr.io
docker build -t $DOCKER_IMAGE .
… and push it to Azure Container Registry
az acr login --name $ACR_NAME
docker tag $DOCKER_IMAGE $ACR_SERVER/$DOCKER_IMAGE
docker push $ACR_SERVER/$DOCKER_IMAGE
Once this is done, you can confirm using az acr repository list
az acr repository list --name $ACR_NAME --output table
Deploy to Kubernetes
To deploy and confirm
kubectl apply -f kstreams-count-statefulset.yaml
kubectl get pods -l=app=kstreams-count
The app will take some time to start up since this also involves storage (Azure Disk) creation and attachment. After some time, you should see two pods in the Running
state
The moment of truth!
It’s time to test our end to end flow. Just to summarize:
- you will produce data to the input Kafka topic (
input-topic
) using the Kafka CLI locally
- the stream processing application in AKS will churn the data, store state and put it back to another Kafka topic
- your local Kafka CLI based consumer process will get that data from the output topic (
counts-topic
)
Let’ create the Kafka topics first
export KAFKA_HOME=[kafka installation directory]
export INPUT_TOPIC=input-topic
export OUTPUT_TOPIC=counts-topic
$KAFKA_HOME/bin/kafka-topics.sh --create --topic $INPUT_TOPIC --partitions 4 --replication-factor 1 --bootstrap-server $KAFKA_BROKER
$KAFKA_HOME/bin/kafka-topics.sh --create --topic $OUTPUT_TOPIC --partitions 4 --replication-factor 1 --bootstrap-server $KAFKA_BROKER
$KAFKA_HOME/bin/kafka-topics.sh --list --bootstrap-server $KAFKA_BROKER
Start consumer process
export KAFKA_HOME=[kafka installation directory]
export KAFKA_BROKER=[kafka broker e.g. localhost:9092]
export OUTPUT_TOPIC=counts-topic
$KAFKA_HOME/bin/kafka-console-consumer.sh --bootstrap-server
$KAFKA_BROKER --topic $OUTPUT_TOPIC --from-beginning --property "print.key=true"
Start producer process (different terminal)
export KAFKA_HOME=[kafka installation directory]
export KAFKA_BROKER=[kafka broker e.g. localhost:9092]
export INPUT_TOPIC=input-topic
$KAFKA_HOME/bin/kafka-console-producer.sh --broker-list $KAFKA_BROKER --topic $INPUT_TOPIC
You will get a prompt and you can start entering values e.g.
> foo:bar
> hello:world
> hello:universe
> foo:baz
> john:doe
In the consumer terminal, you should see the words and their respective counts e.g. foo 2
, hello 2
, john 1
etc.
With the sanity testing out of the way…
… let’s look at the state of AKS cluster.
Check the PersistentVolumeClaim
s (PVC) and PersistentVolume
s (PV) – you will two separate set of PVC-PV pairs.
kubectl get pv
kubectl get pvc
The creation of PersistentVolume
s means that Azure Disks were created as well. To check them, let’s get AKS node resource group first
AKS_NODE_RESOURCE_GROUP=$(az aks show --resource-group abhishgu-aks --name abhishgu-aks --query nodeResourceGroup -o tsv)
Assuming there this is a two node AKS cluster we will get back four disks – one each for the two nodes and one each of for two of our app instances
az disk list -g $AKS_NODE_RESOURCE_GROUP
You will notice that name of disk is the same as that of the PVC
Let’s dig into the file system of the Pod
which is running our application. kstreams-count-0
is the name of one such instance (yes, the name is deterministic, thanks to StatefulSet
). Recall that we specified /data/count-store
as the state store directory in our app as well the as volumeMounts
section of the app manifest – let’s peek into that directory.
kubectl exec -it kstreams-count-0 -- ls -lrt /data/count-store/counts-app
You will notice that the state data split across multiple sub-directories whose number will be equal to the number of topic partitions which the app instance is handling e.g. if you have four partitions and two instances, each of them will handle data from two partitions each
total 24
drwxr-xr-x 3 root root 4096 Sep 16 11:58 0_0
drwxr-xr-x 3 root root 4096 Sep 16 12:02 0_1
you can repeat the same process for the second instance i.e. kstreams-count-1
If you list the number of topics using the Kafka CLI, you should also see a topic named counts-app-counts-store-changelog
. This is the back-up, log-compacted changelog topic which we discussed earlier
the name format is --changelog
Clean up
Start by deleting the StatefulSet
and associated Headless
Service
kubectl delete -f kstreams-count-statefulset.yaml
The PersistentVolume
s associated with the PersistentVolumeClaim
s are not deleted automatically
kubectl delete pvc
This will trigger the deletion of the PersistentVolume
s and the corresponding Azure Disks. You can confirm the same
kubectl get pv
az disk list -g $AKS_NODE_RESOURCE_GROUP
Finally, to clean up your AKS cluster, ACR instance and related resources
az group delete --name $AZURE_RESOURCE_GROUP --yes --no-wait
That’s all for this blog! If you found it helpful, please like and follow 🙂